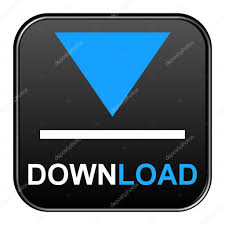
Not explicitly specified for the MatMul operation, the TensorFlow runtime willĬhoose one based on the operation and available devices ( GPU:0 in thisĮxample) and automatically copy tensors between devices if required. You will see that now a and b are assigned to CPU:0. To create a device context, and all the operations within that context will
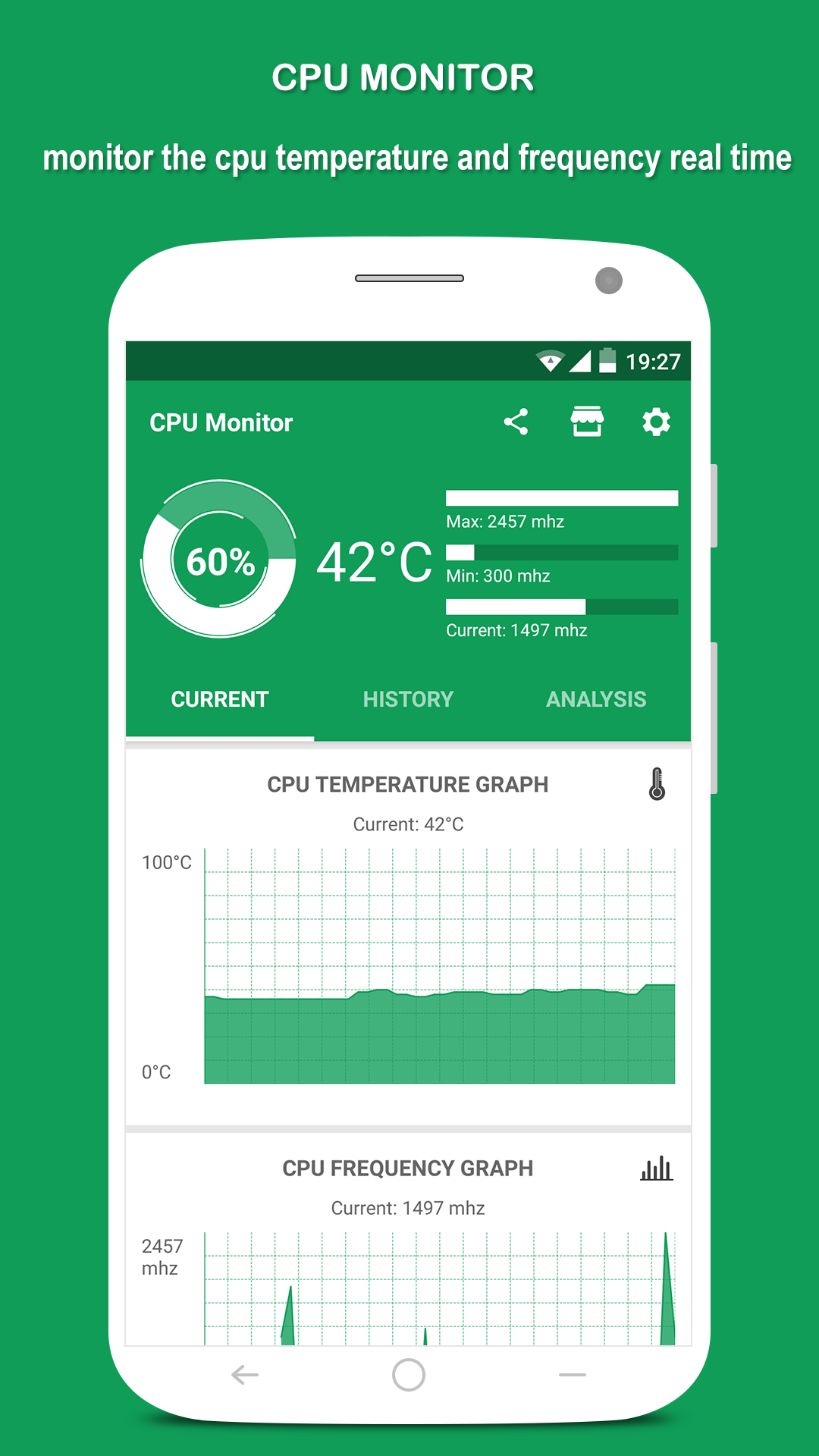
Instead of what's automatically selected for you, you can use with tf.device If you would like a particular operation to run on a device of your choice
Cpu gpu memory monitor code#
The above code will print an indication the MatMul op was executed on GPU:0. tf.t_log_device_placement(True)Ī = tf.constant(, ])ī = tf.constant(,, ])Įxecuting op _EagerConst in device /job:localhost/replica:0/task:0/device:GPU:0Įxecuting op MatMul in device /job:localhost/replica:0/task:0/device:GPU:0 Enabling device placement logging causes any Tensor allocations or operations to be printed. Tf.t_log_device_placement(True) as the first statement of your To find out which devices your operations and tensors are assigned to, put For example, since tf.cast only has a CPU kernel, on a system with devices CPU:0 and GPU:0, the CPU:0 device is selected to run tf.cast, even if requested to run on the GPU:0 device. If a TensorFlow operation has no corresponding GPU implementation, then the operation falls back to the CPU device. For example, tf.matmul has both CPU and GPU kernels and on a system with devices CPU:0 and GPU:0, the GPU:0 device is selected to run tf.matmul unless you explicitly request to run it on another device. If a TensorFlow operation has both CPU and GPU implementations, by default, the GPU device is prioritized when the operation is assigned. "/job:localhost/replica:0/task:0/device:GPU:1": Fully qualified name of the second GPU of your machine that is visible to TensorFlow."/GPU:0": Short-hand notation for the first GPU of your machine that is visible to TensorFlow.

"/device:CPU:0": The CPU of your machine.They are represented with string identifiers for example: TensorFlow supports running computations on a variety of types of devices, including CPU and GPU. If you would like to use Nvidia GPU with TensorRT, please make sure the missing libraries mentioned above are installed properly. 02:52:22.648933: W tensorflow/compiler/tf2tensorrt/utils/py_:38] TF-TRT Warning: Cannot dlopen some TensorRT libraries. 02:52:22.648924: W tensorflow/compiler/xla/stream_executor/platform/default/dso_:64] Could not load dynamic library 'libnvinfer_plugin.so.7' dlerror: libnvinfer_plugin.so.7: cannot open shared object file: No such file or directory 02:52:22.648829: W tensorflow/compiler/xla/stream_executor/platform/default/dso_:64] Could not load dynamic library 'libnvinfer.so.7' dlerror: libnvinfer.so.7: cannot open shared object file: No such file or directory Print("Num GPUs Available: ", len(tf.config.list_physical_devices('GPU'))) SetupĮnsure you have the latest TensorFlow gpu release installed.
Cpu gpu memory monitor how to#
To learn how to debug performance issues for single and multi-GPU scenarios, see the Optimize TensorFlow GPU Performance guide. This guide is for users who have tried these approaches and found that they need fine-grained control of how TensorFlow uses the GPU. The simplest way to run on multiple GPUs, on one or many machines, is using Distribution Strategies. Note: Use tf.config.list_physical_devices('GPU') to confirm that TensorFlow is using the GPU.
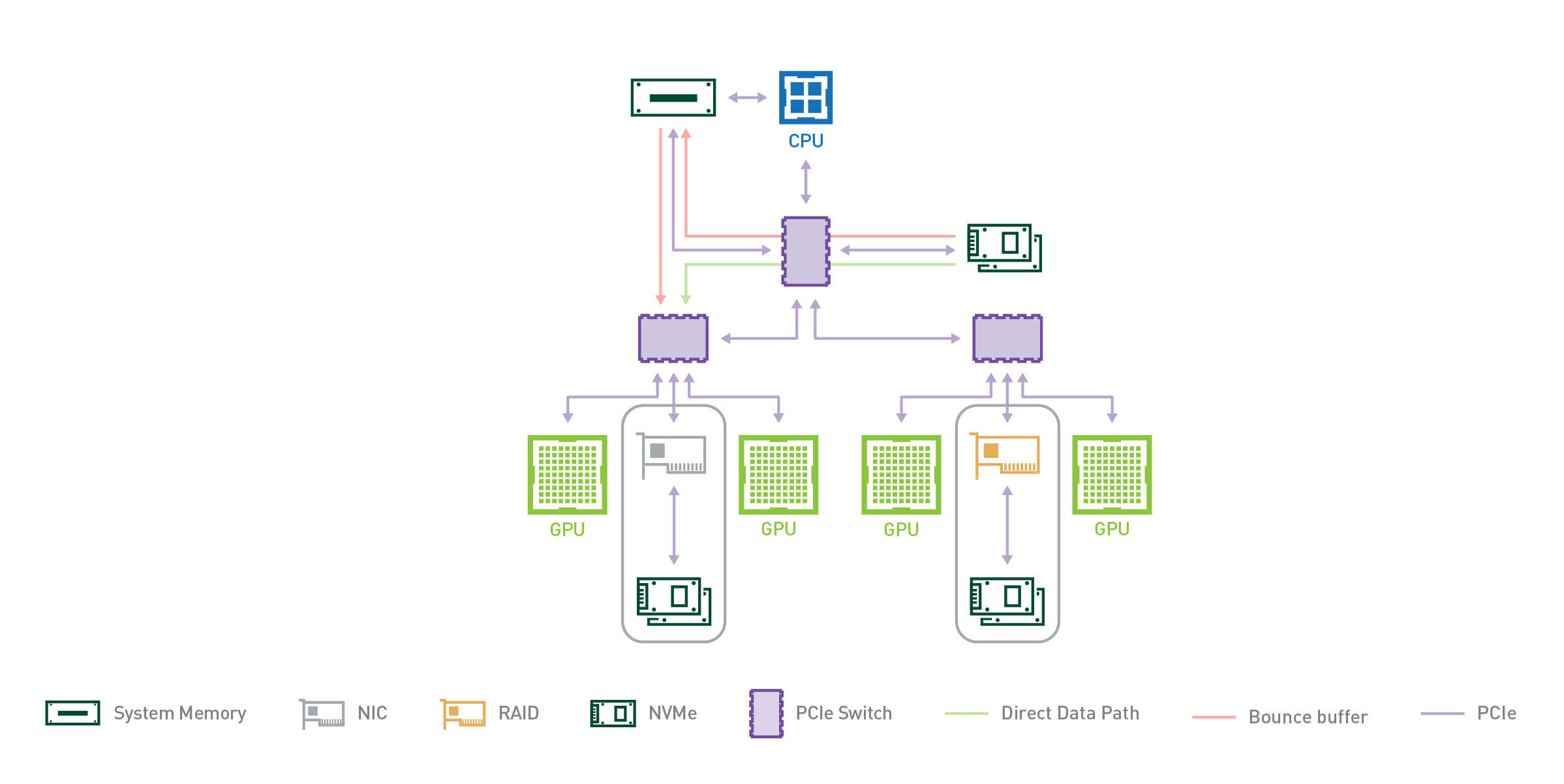
TensorFlow code, and tf.keras models will transparently run on a single GPU with no code changes required.
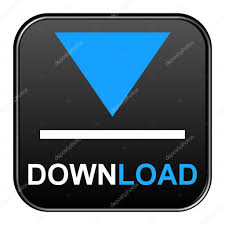